Effects of Big Data Management on Industrial Growth: A Case for the Organization of Economic Cooperation and Development Countries
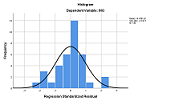
Abstract:
Industrial
growth is an essential condition for sustainable economic development. However,
data management also plays an important role in ensuring effective planning and
result-oriented decision-making in an organization. Although big data management
is essential in this regard, its usage in most countries seems to be a new field.
The aim of this study was to examine the effect of big data management on industrial
growth in the Organization of Economic Cooperation and Development (OECD) countries.
The study used expost-facto design approach and time-series or secondary data covering
2018 to 2020. A sample of 43 countries were used for the study. The Ordinary Least
Square (OLS) regression technique was used as a technique for data analysis. The
results from the descriptive analysis revealed that ICT access and usage had a higher
mean value than internet access which signifies that ICT access and usage contributed
more to industrial growth in OECD than internet access (INA). The findings from
the analysis of the hypotheses also found that ICT access and usage and internet
access have a significant effect on industrial growth in OECD countries. The study,
therefore, concluded that big data management had positive effects on industrial
growth in OECD countries and recommended that governments of OECD countries should
invest more on internet access so as to promote efficiency in big data management
and that they should also provide ICT infrastructure that are necessary for effective
management of big data and industrial growth.
References:
[1] Ware, B. (2018). Top 10 data management challenges: Availability and
security https://www.progress.com/blogs/top-10-data-management-challenges-availabilitysecurity/.
[2] Crawford, M (2016).
Making sense of sensors. https://www.asme.org/topics-resources/content/making-sense-of-sensors.
[3] Belyh, A. (2019). Brief history
of big data. https://www.cleverism.com/brief-history-big-data.
[4] Bulao, J., (2021). How much data is created every day in 2021?
https://techjury.net/blog/how much-data-is-created-every-day/.
[5] Connall, M. (2021). Top
20 big data statistics for 2021. https://www.sigmacomputing.com/blog/top-20-big-data-statistics/.
[6] Arora,V., (2018). How big data influences the decision making for
enterprises? https://thenextscoop.com/big-data-influences-decision-making/.
[7] Forbes, (2019). 95
percent of businesses need to manage unstructured data. https://www.dataideology.com/data/95-percent-of-businesses-need-to-manage-unstructured-data.
[8] Vaghela, Y. (2018). Four common big data challenges.
https://www.dataversity.net/four-common-big-data-challenges/.
[9] Lynkova, D., (2019). 39+ big data statistics for 2020.
https://leftronic.com/big-data-statistics/Madeline.
[10]Statistical
Commission (2016). Report on the global working
group on big data for official statistics. Vienna: UNIDO.
[11]
Bahrini,
R. & Qaffas, A. A., (2019). Impact of information and communication technology
on economic growth: Evidence from developing countries. Economies 7(21), 1-13. Doi:10.3390/economies7010021.
[12] Rai, A. (2020). What is big data: Characteristics, types,
benefits and examples. https://www.upgrad.com/blog/what-is-big-data-types-characteristics-benefits-and-examples.
[13]
Slideshare, (2015). Big data unit. https://www.slideshare.net/navjotkaur313371/big-data-unit-i.
[14] Big Data Framework
(2019). Where
does ‘big data’ come from? https://www.bigdataframework.org/short-history-of-big-data/.
[15]
Gallagher, B. (2018). The top 5 factors to consider when using big
data technology. https://www.ispartnersllc.com/blog/top-5-factors-using-big-data/.
[16] Galov,
N. (2021). 77+big
data statistics for the big future ahead. https://hostingtribunal.com/blog/big-data-stats/.
[17]
Priceonomics (2020). Companies collect a lot of data, but how much do. They actually use?
https://priceonomics.com/companies-collect-a-lot-of-data-but-how-much-do/.
[18] Splunk, H. (2019). The state of dark data. www.splunk.com/pdfs/dark-data/the-state-of-dark-
data-report.pdf.
[19] Johnson,
J., (2020). What is dark data?
The basics and the challenges. https://www.progress.com/blogs/top-10-data-management-challenges-availability-security.
[20] Sorbe,
S. (2019). Digital dividend: Policies to harness
the productivity potential of digital technologies. OECD Economic Policy Paper,
No. 26 Paris: OECD Publishing. https://doi.org/10.1787/273176bc-en.
[21] Tozzi, C., (2020). Big data industries: 5 industries being reshaped by data
analytics. https://www.precisely.com/blog/big-data/big-data-industries-data-analytics.
[22] Rossi, R., & Hirama,
K. (2015). Characterizing Big Data Management. Issues in Informing Science and Information
Technology, 12, 165-180. http://iisit.org/Vol12/IISITv12p165-180Rossi1921.pdf.
[23]
Harvey, C. (2017). Big data challenges. https://www.datamation.com/big-data/big-data-challenges/Yuvrajsinh.
[24] Bekker, A. (2018).
The ‘scary’ seven: big data challenges and
ways to solve them https://www.bigdataframework.org/formulating-a-big-data-strategy.
[25] Sharma, R., (2020). Top 6 major challenges
of big data & simple solutions to solve them. https://www.upgrad.com/blog/major-challenges-of-big-data.
[26] Andrews,
D. & Criscuolo, C., (2013). Knowledge-based capital, innovation and resource
allocation”. OECD Economics Department Working
Papers, No. 4. Paris: OECD Publishing https://doi.org/10.1787/5k46bh92lr35-en.
[27] Organization of Economic Cooperation and Development (OECD) (2019), ICT access and usage by businesses. https://stats.oecd.org/Index.aspx?DataSetCode=ICT_BUS accessed on 18 February 2021.
[28] Haskel, J. & Westlake’s., (2017). Capitalism without capital: the rise of the intangible economy. Princeton: Princeton University Press. http://www.pse-journal.hr/downloadpdf. php articleId=5434.
[29] Brynjolfsson,
E., D. Rock and C. Syverson (2017). Artificial intelligence and the modern productivity
paradox: A clash of expectations and statistics. NBER working paper 24001. Cambridge:
National Bureau of Economic Research. https://www.nber.org/system/files/working_papers/w.
[30] Calvino,
F. (2018). A taxonomy of digital intensive sectors. OECD science, technology and industry working papers, No. 2018/14.Paris:
OECD Publishing http://dx.doi.org/10.1787/f404736a-en.